Seminar: Probabilistic Commonsense Knowledge in Language
Lorraine (Xiang) Li
UMass Amherst
Wednesday, March 16, 2022
10:00 AM
1100 Torgersen Hall
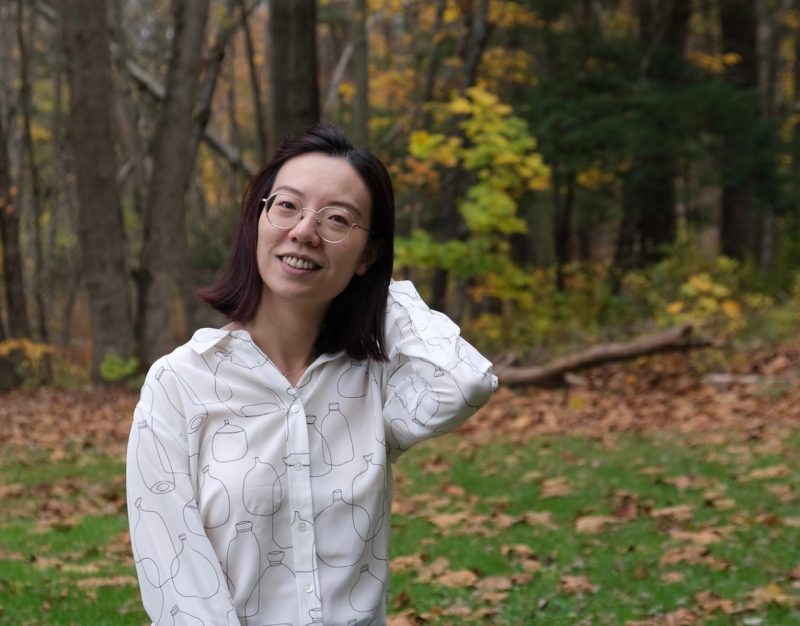
Abstract
Commonsense knowledge is critical to achieving artificial general intelligence. This shared common background knowledge is implicit in all human communication, facilitating efficient information exchange and understanding. However, commonsense research is hampered by its immense quantity of knowledge because an explicit categorization is impossible. Furthermore, a plumber could repair a sink in a kitchen or a bathroom, indicating that common sense reveals a probable assumption rather than a definitive answer. To align with these properties of commonsense fundamentally, we want to model and evaluate such knowledge human-like using probabilistic abstractions and principles.
This talk will introduce a probabilistic model representing commonsense knowledge using a learned latent space of geometric embeddings -- probabilistic box embeddings. Using box embeddings makes it possible to handle commonsense queries with intersections, unions, and negations in a way similar to Venn diagram reasoning. Meanwhile, existing evaluations do not reflect the probabilistic nature of commonsense knowledge. To fill in the gap, I will discuss a method of retrieving commonsense related question answer distributions from human annotators and a novel method of generative evaluation. We utilize these approaches in two new commonsense datasets (ProtoQA, Commonsense frame completion). The combination of modeling and evaluation methods based on probabilistic principles sheds light on how commonsense knowledge can be incorporated into artificial intelligence models in the future.
Biography
Lorraine (Xiang) Li is a final-year Ph.D. candidate at UMass Amherst working with Andrew McCallum. Her research focuses on designing probabilistic models and evaluation methods for implicit commonsense knowledge in language. Her research is at the intersection of natural language processing, commonsense reasoning, knowledge representation, and machine learning. During her Ph.D., she interned at multiple companies, including Google, Bloomberg, Facebook (now Meta) AI Research, and DeepMind. Previously, she obtained an M.S. in Computer Science from The University of Chicago while conducting NLP research at TTIC.